CS224n Lecture 14 Transformers and Self-Attention For Generative Models
14강은 강연자를 초대해서 강의를 진행한다. Google AI에서 나온 연사자 두분이라고 한다. NLP 공부하려고 듣는 것이고, 다른 것들 하기에도 약간 벅찬듯 싶어서 NLP 내용을 좀 벗어나는 이미지 처리, 음성 처리 같은 부분은 많이 건너뛰었다 ㅠㅠ
Previous works
Variable Length Data의 representation을 학습하는 것은 NLP에서 매우 중요하다. 그를 위한 선택지를 여러가지 꼽을 수 있는데, 우선 RNN은 Variable Length Representation을 학습하기 위한 좋은 선택지이고, LSTM, GRU같은 검증된 모델이 나와있지만, Sequential Computation을 해야하기 때문에 병렬화가 어렵고, long, short range dependency에 대한 모델링이 어렵다. 그래서 병렬화가 쉬운 CNN을 이용하면 long dependency를 학습하기가 매우 어려워진다. layer를 엄청 쌓아야지만 학습이 가능해진다. NMT의 Encoder와 Decoder사이에서 좋은 성능을 보인 Attention같은 경우는 Representation에도 사용하는 것이 어떤지에 대한 아이디어가 나왔고 좋은 성능을 보였다고 한다. 주로 언급되는 모델은 self-attention.
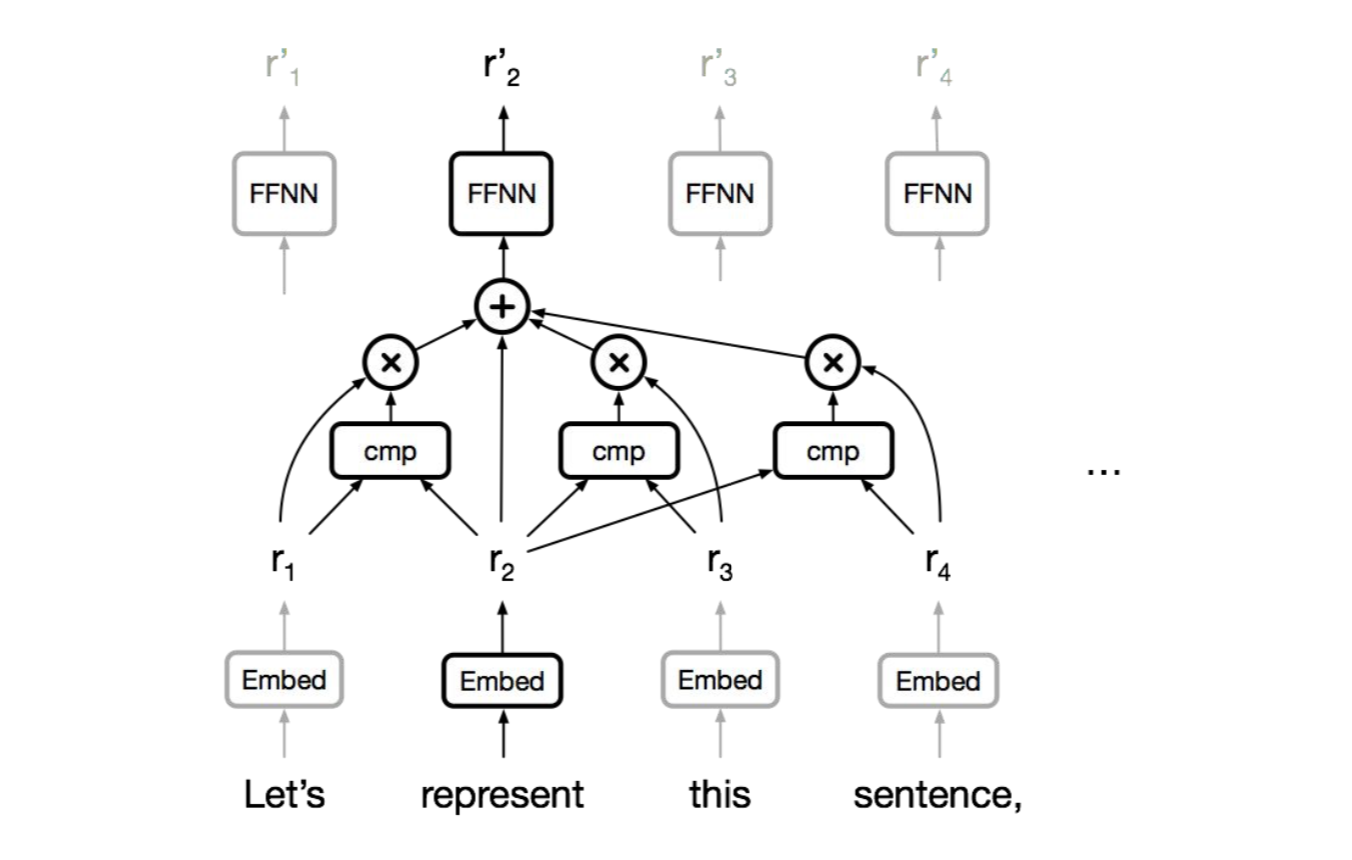
self attention에서는 short dependency던, long dependency던 constant path length를 준다고 한다. 그리고 gating/multiplicative interaction이 기반인 모델이다. (matmul 같은) “그럼 이 모델이 sequential computation을 대체할 수 있을까?”라는 질문이 자연스레 나오게 되고, 그에 대한 대답이 Transformer이다.
추가적으로 살펴볼 수 있는 자료들은 아래와 같다.
- Classification & regression with self-attention: Parikh et al. (2016), Lin et al. (2016)
- Self-attention with RNNs: Long et al. (2016), Shao, Gows et al. (2017)
- Recurrent attention: Sukhbaatar et al. (2015)
Transformer
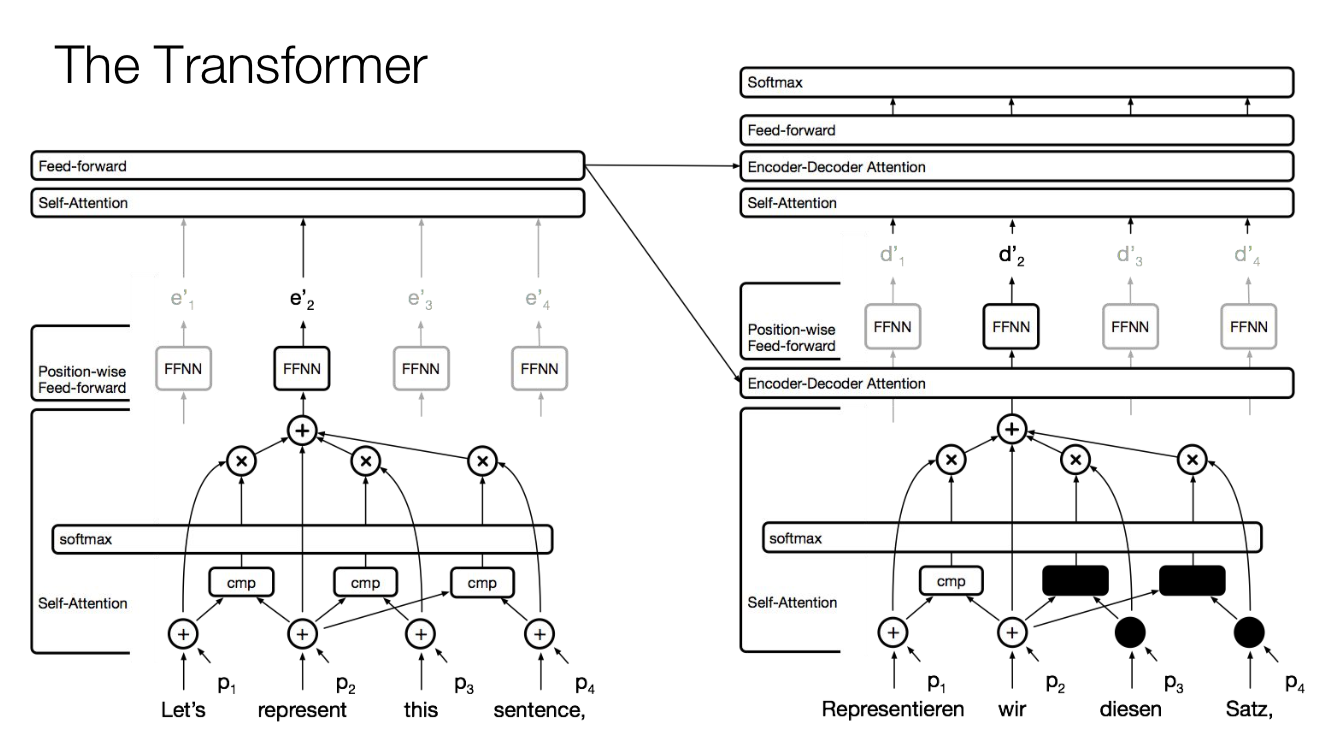
residual connection, self-attention layer 같은 이전의 강의에 설명이 되었던 부분에 대해 전체적으로 설명을 하면서 시작한다. 오른쪽 아래 레이어의 attention 일부분이 보이지 않는 이유는 masked multi-head attention layer이기 떄문이다. (Attention is All You Need 논문 참고)
Attention is Cheap
Self Attention의 computational complexity는 \(O(length^2 * dim)\)인데, RNN의 computation complexity는 \(O(length * dim^2)\)이다. 따라서 length가 dim보다 작은 상황에서 훨씬 적어진다. 강의에서 나온 LSTM의 상황은 length와 dim이 같더라도 4배나 적은 complexity를 가진다.
Convolution vs Attention vs Multihead Attention
하지만 Attention은 문제점이 있는데, 예를 들어 I kicked the ball
이라는 문장에 대해서 convolution을 진행한다고 하면, 긱긱의 단어에 filter가 다른값을 적용하면서 필요한 값을 뽑아낸다. 하지만, Attention은 그를 averaging하므로, 필요한 정보를 뽑아내기가 힘들다. 그래서 multi-head attention이 나왔다. 그래서 필요한 정보만을 적당히 뽑아낼 수 있게 된다.
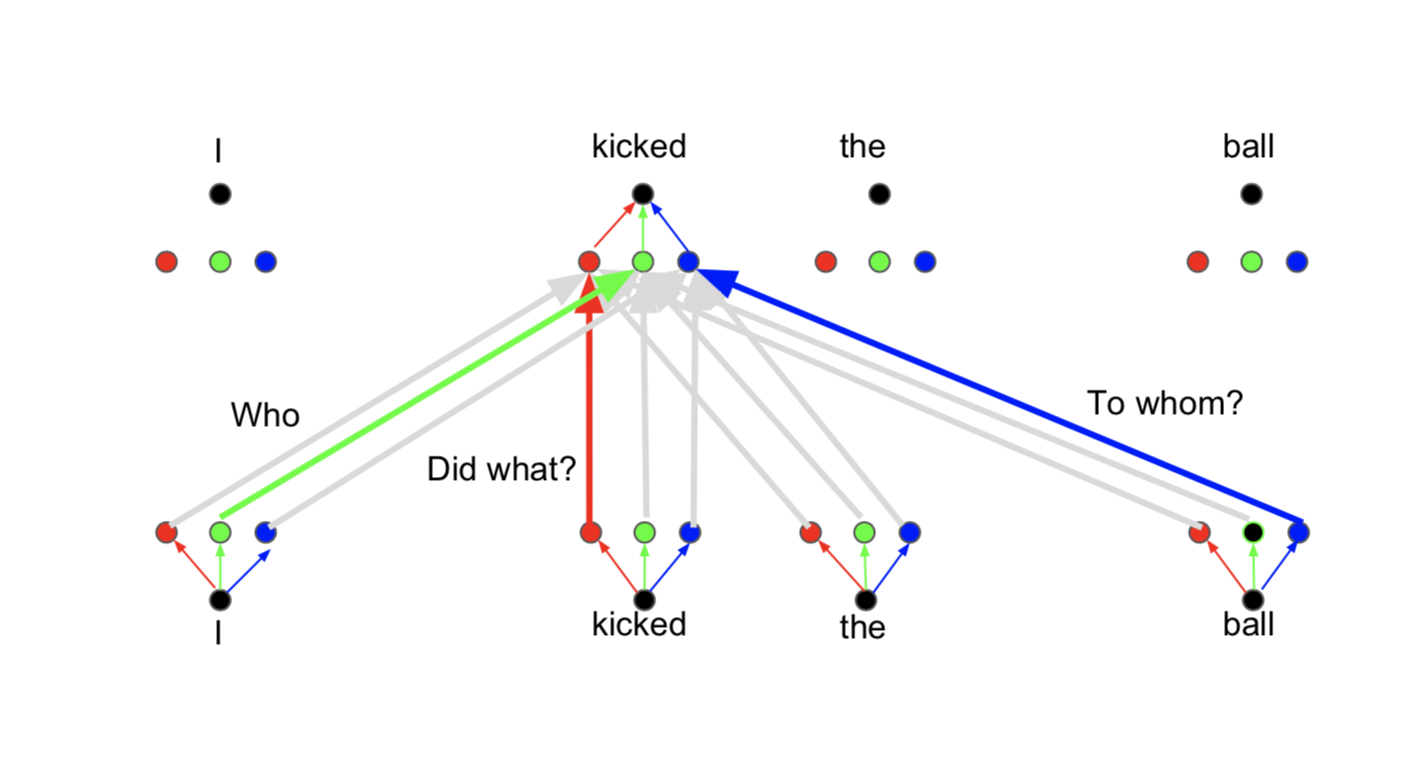
Results
너무 좋은 성능을 보이고, SOTA도 많이 찍으니까 최근에 많은 모델들이 transfomer 기반으로 나온다. framework들은 tensor2tensor1, Sockeye2를 찾아보자.
Importance of Residual Connections
Residual connection을 이용하면 positional information을 higher layer로 다른 정보와 함께 전달해줄 수 있다고 한다.
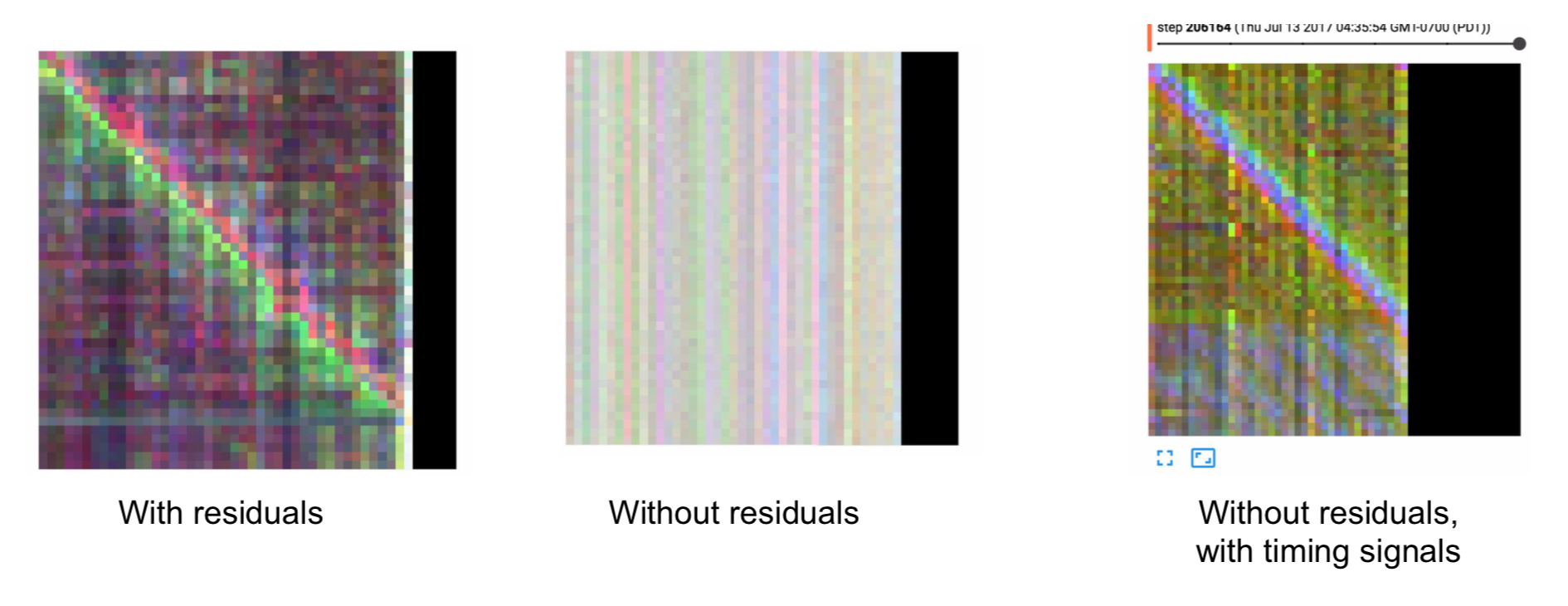
_
Attention을 이용하는 만큼 이용할 수 있는 Transfer Learning과 같은 키워드를 찾아봐도 좋을 것 같다. (아직 이해 잘 못함) 뭔가 소개하는 것을 위주로 쭈우욱 지나갔는데 너무 빨리 쭉 지나가서 흥미로운 내용도 많았지만, 제대로 캐치를 못한 것 같아서 CS224n 스터디가 끝나고 나면 이 강의만 다시 보아도 좋을 것 같다.
-
github tensorflow/tensor2tensor tensor2tensor repository ↩
-
github awslabs/sockeye sockeye repository ↩