CS224n Lecture 17 The Natural Language Decathlon: Multitask Learning as Question Answering
Richard Socher라는 Saleforce의 Chief Scientist가 게스트로 나와 강의를 한다고 한다.
강의는 전체적으로 multi-task learning에 대한 내용인데, single-task의 한계에 대해서 먼저 알아보자. 최근에 dataset, task, model, metric에 대한 엄청난 발전이 있었지만, 예전에는 새로운 모델은 거의 random한 상태에서 새로 시작하거나 일부만 pre-train된 상태에서 시작해야했다. 하지만 시간이 지나면서 word2vec, GloVe, CoVe, ELMo, BERT처럼 더 많은 부분을 pretrain해서 모델을 새로 구성할 때 더 좋은 결과를 내는 것을 볼 수 있었다.
그럼 전체를 왜 pretrained model을 사용하지 않을까?
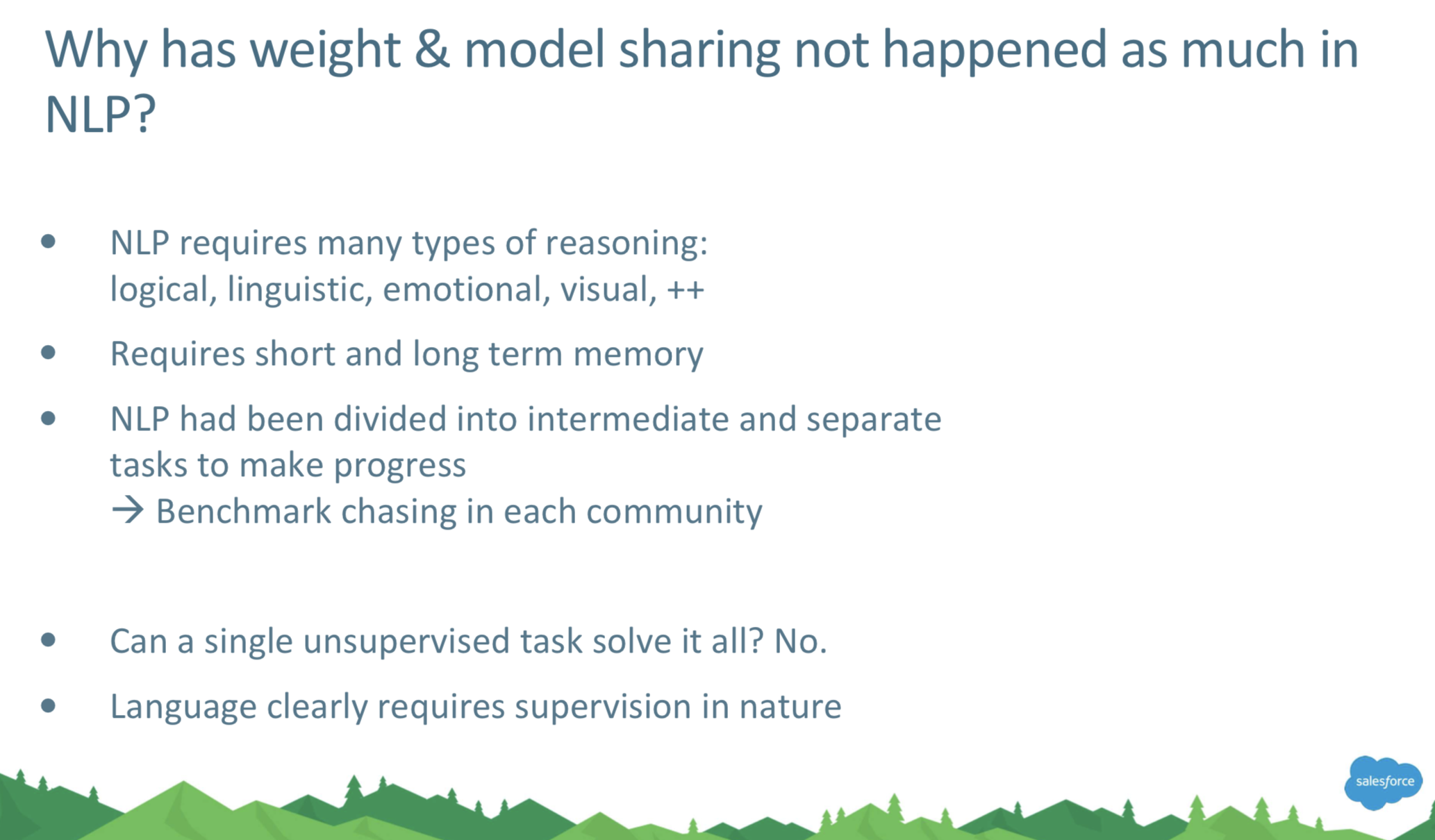
그럼 많은 태스크를 하나의 NLP 프레임워크로 묶을 수는 없을까?
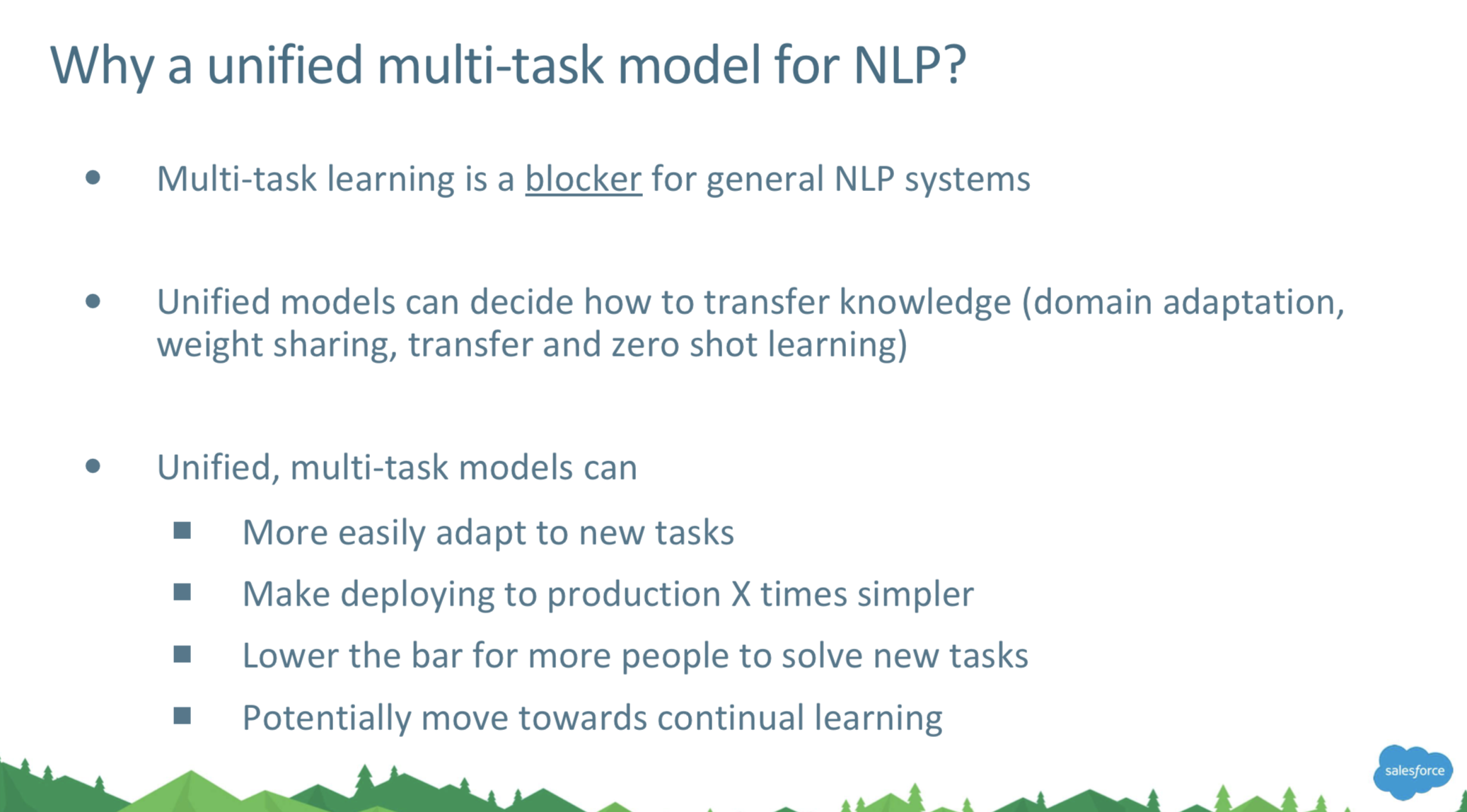
그럼 크게 3개의 분류로 NLP 태스크들을 나누어보자
- sequence tagging : NER, aspect specific sentiment
- text classification : dialogue state tracking, sentiment classification
- seq2seq : MT, summarization, QA
결론은 salesforce에서 개발하고 있는 decaNLP에 대한 약간의 홍보가 들어가기도 하는 것 같지만, 어쨌든 이런 multitask Learning을 목표로 하고 개발한 시스템이라고 한다. decaNLP는 task-specific한 module이나 parameter가 없다고 한다. 하지만 여러개의 다른 태스크를 수행하기 위해 조정이 가능하다고 한다. 보지못한 태스크에 대해서 대응하고 싶었다고.
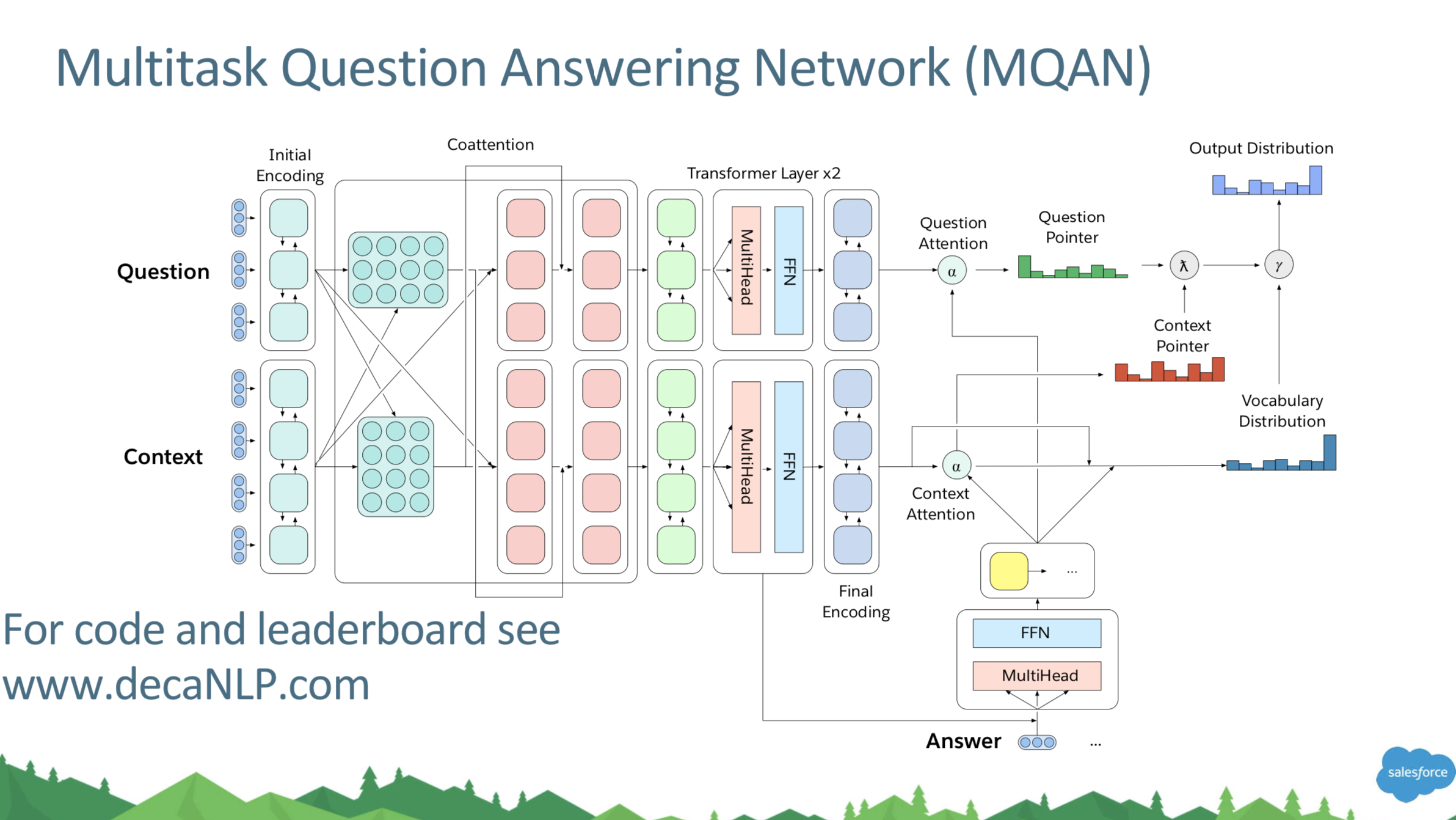
그리고 multitask QA에 대해 설명해주는데 완전 재밌어보인다. fixed GloVe + character n-gram embedding으로 linear layer 거친 후에 Shared BiLSTM + skip connection으로 연결한거 거치고 attention summation해주는 부분이 있는데 이 부분 제대로 이해못했다. 왜 그렇게 하는지..? 암튼 서로 attention을 잘 섞어주고 나서 차원 축소를 위해 또 BiLSTM을 거친 후 Transformer Layer를 거친다. 그리고 Transformer layer 이후로 제대로 이해 못함..
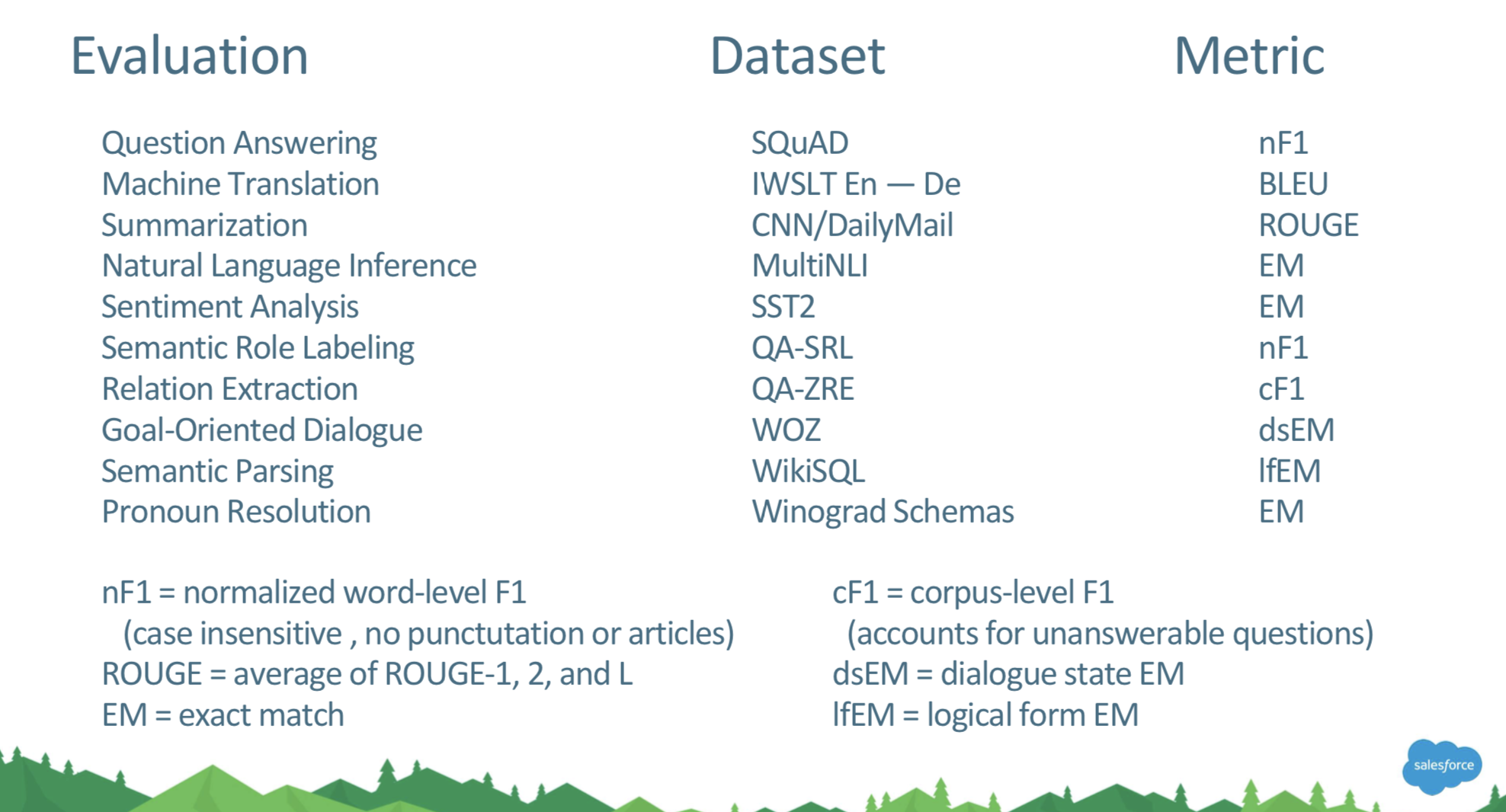
태스크별로 데이터셋 - Metric은 이렇게 사용했다고 한다.
그 다음에는 multitask learning을 위한 training strategy를 설명해준다. 첫번째는 fully joint.
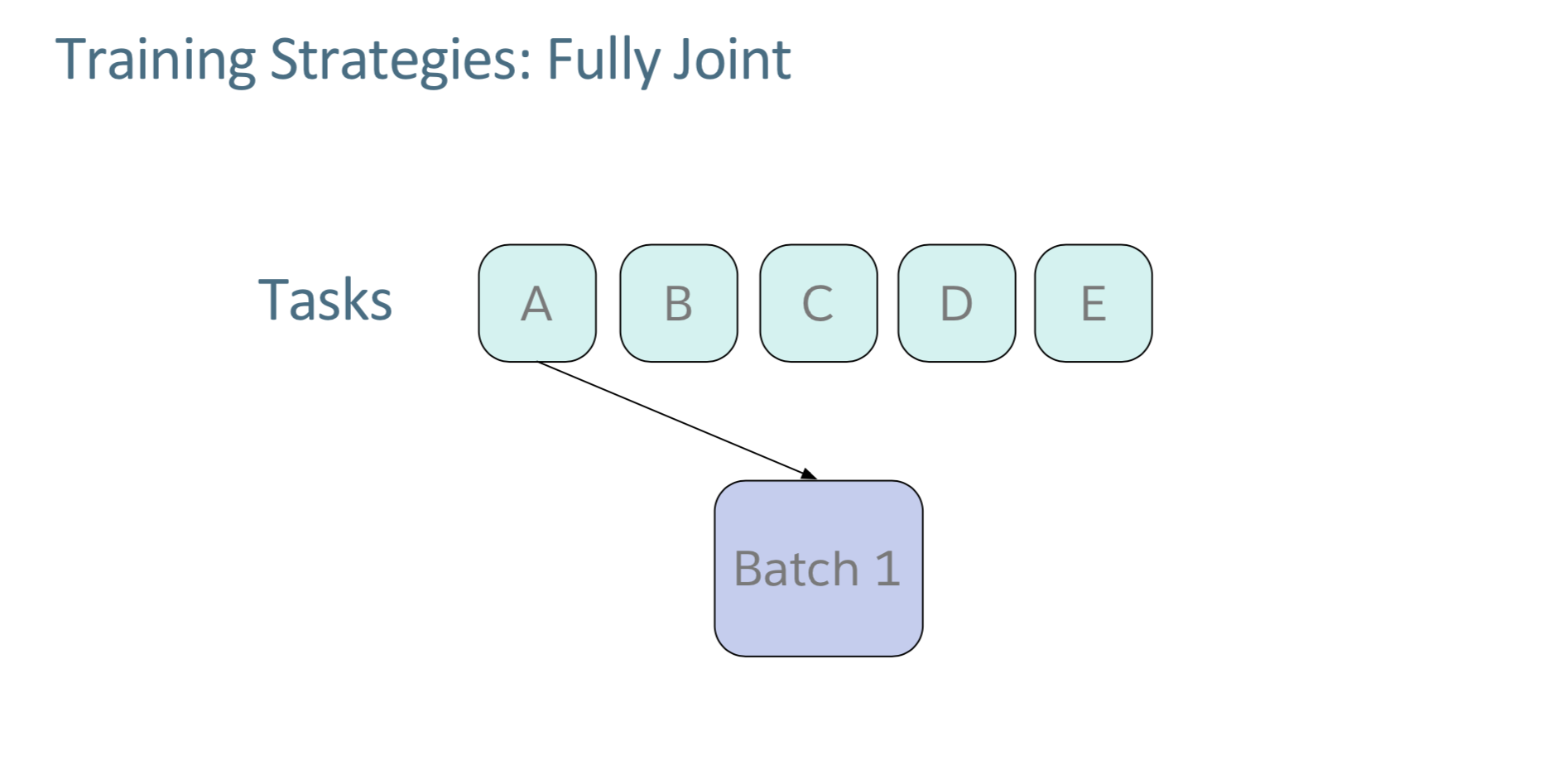
The first strategy we consider is fully joint. In this strategy, batches are sampled round-robin from all tasks in a fixed order from the start of training to the end. This strategy performed well on tasks that required fewer iterations to converge during single-task training (see Table 3), but the model struggles to reach single-task performance for several other tasks. In fact, we found a correlation between the performance gap between single and multitasking settings of any given task and number of iterations required for convergence for that task in the single-task setting.
강의 설명을 잘 이해하지 못하곘어서 해당 논문을 찾아보았다. curriculum learning1을 위처럼 논문에서 설명하는데, batch를 sampling할 때, fixed order로 계속 RR로 돌면서 수집하는 것이라고 한다. 엄청 많이 돌아가 converge되는 태스크들은 잘 동작하지 않는다고. 그래서 anti-curriculum learning을 시도해보았다고 하는데, 이거는 phase를 두개로 나눈 다음에 첫번째는 jointly하게 학습하고 보통 이들이 더 어려운 것들이라고 한다. 두번째 페이즈는 fully jointly로 학습한다.
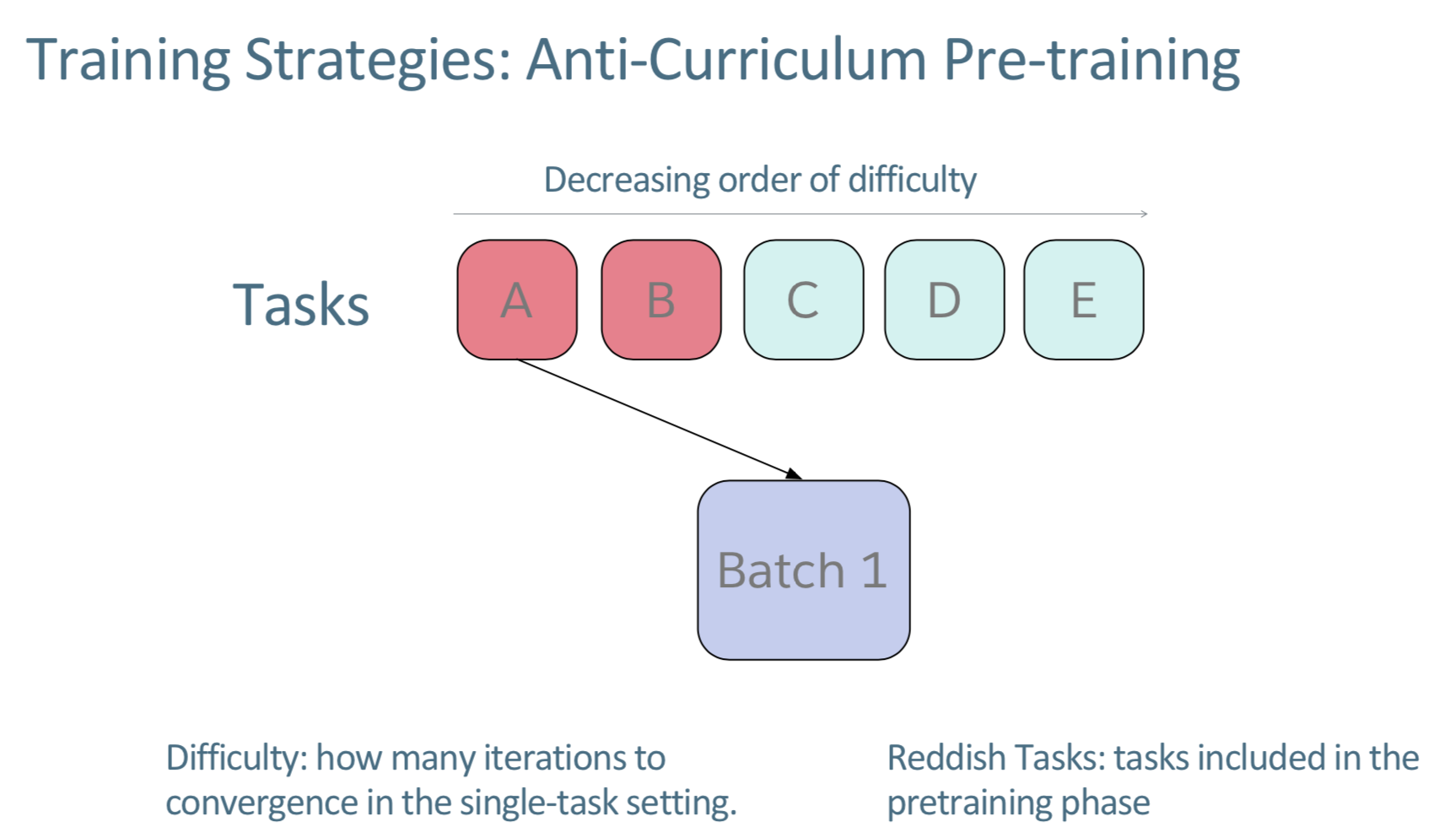
빨강이 first phase이고, 파랑이 그 나머지이다. Reddish한 부분이 어렵고 반대쪽이 쉽다고.
암튼 그 결과에 대해서 열심히 말하다가 끝내는데, Related Work를 더 많이 읽어보아야 앞 부분도 잘 이해할 듯 싶다
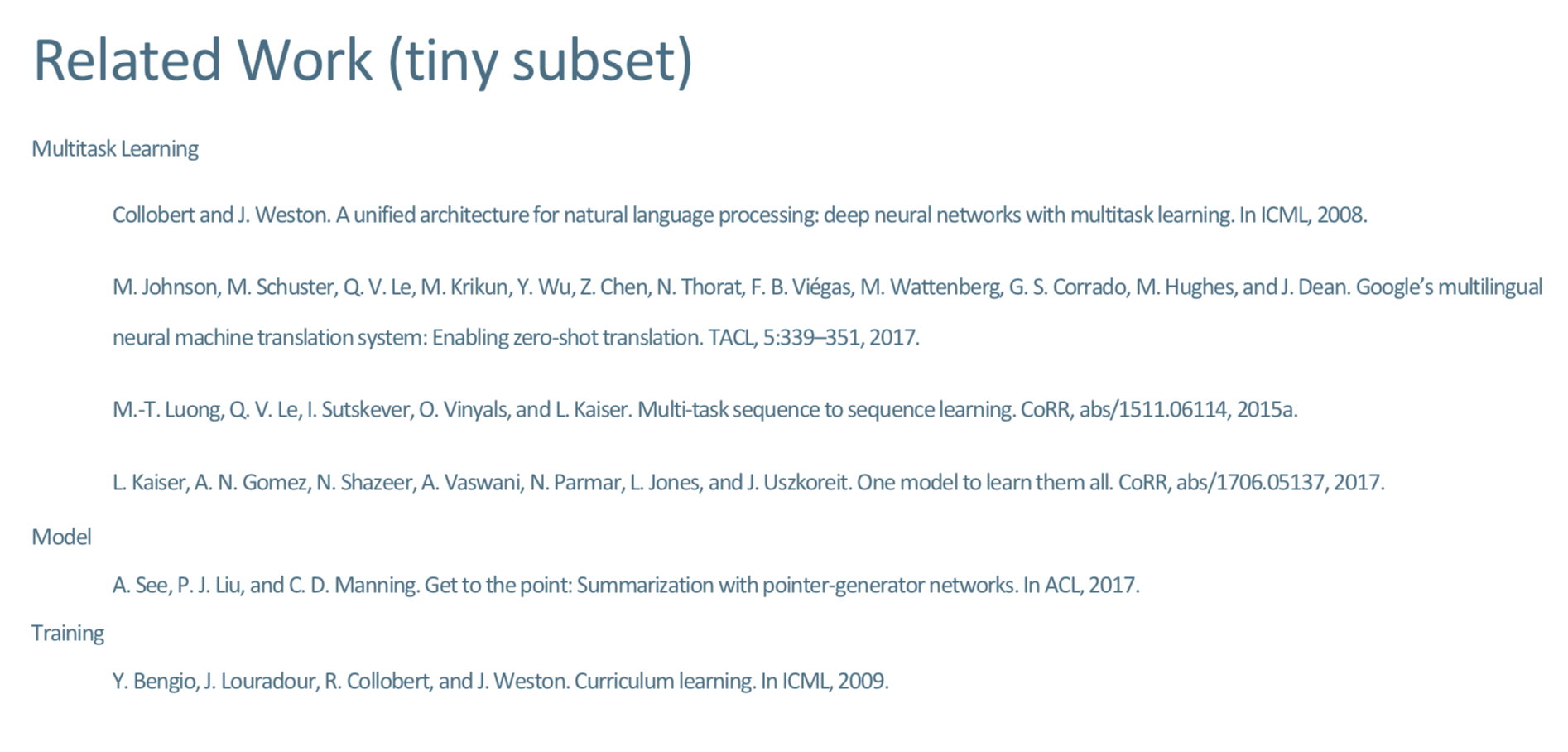
위 목록을 읽어보자..